Major US insurance provider chooses comforte for NACHA compliance
Download- Fulfilled latest data protection requirements from NACHA and prepared for future regulations
- Enabled data scientists to more effectively detect fraud without exposing PII
- Automated and agentless data discovery for highly efficient data governance
- Cloud native security enabling transformation to DevOps methodology
- Solution implemented in a fraction of the time that competing solutions demand
This Fortune 500 health insurance provider is among the largest and fastest growing property/casualty insurance groups in the United States. In addition to P&C, they cover life insurance, annuities, retirement investment, homeowners, automotive, and workers’ compensation lines in addition to advisory and investment services.
They have a complex data environment with a mix of cloud and on-premises applications and are in the process of transforming to cloud native DevOps. Their core claims application runs on-premises with millions of transactions daily while interacting with dozens of other applications. Due to their rapid growth strategy, mergers and acquisitions have resulted in a complex architecture with a multitude of different databases that they’re trying to harmonize.
This major insurer has a complex data environment with legacy tools and needed a modern data protection solution that would enable them to keep sensitive data protected while they transform to a modern DevOps and cloudfirst approach to IT. Because of rapid expansion propelled by acquisitions, their IT environment is very dynamic and frequently changing. They faced three major challenges in achieving that goal.
NACHA Compliance
In addition to impending data privacy legislation at the State level, a hard deadline was approaching for new NACHA requirements. NACHA is similar to PCI DSS in some ways but goes beyond cardholder data and requires protections for additional forms of personal data unrelated to payment account information.
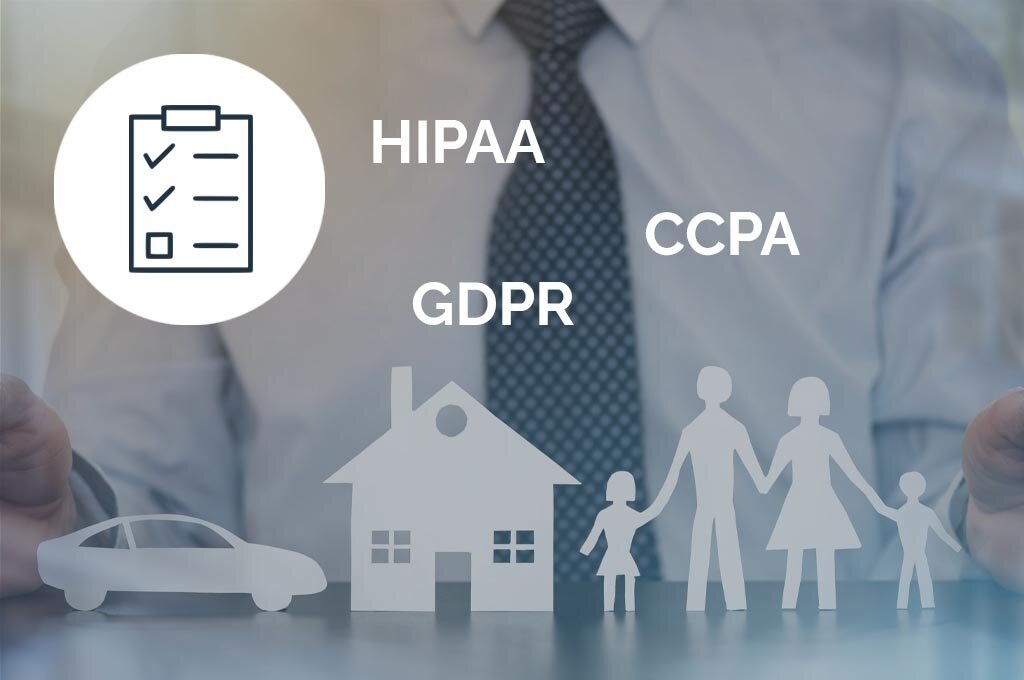
The insurer’s claims processing and quoting system stored millions of records of PII, including driver’s licenses, bank account information, policy information, SSNs, and other sensitive data inside free text fields, both structured and semi structured. While this data was already protected with various means, including an existing tokenization solution, the insurer needed a more agile and scalable solution as they move to cloud based applications for machine learning and analytics. The ability to discover and protect semi-structured sensitive data within free text fields was a key requirement.
Data Analytics, AI, & Machine Learning with Sensitive Data
Their data science team required greater volumes of data for deeper analytics using a blend of Amazon cloud (AWS) and Google BigQuery in the Google Cloud. This required de-risking PII while keeping it in a usable format that allows them to make use of larger data sets.
For example, they had data that was moving operationally from their onpremises ecosystem up into AWS for grooming, then moving upstream into a GCP and Big Query environment for automated analytics processes for insurance risk management, risk reduction, and prediction. With data in motion like this, it was absolutely critical that security traveled with that data throughout the workflow.
The workflow ended with business-critical analysis based in data science, and the entire workflow was geared to achieve hyper-agility while gaining insights. The main challenge here was keeping sensitive data protected but in a state where it could still be used for data analytics with high volume, high velocity, and high variety.
The insurer was working with a legacy ecosystem for traditional claims processing that was patterned on pre-transformation, but the customer was swiftly migrating their applications into the cloud for machine learning and AIbased analysis. Fast-moving data ecosystems have the potential for data exposures which results in an inability to use all the data you need because traditional protection methods can trip up automated machine learning and AI application workstreams.
Unfortunately, their existing data masking solution could not deliver data to the scaled AI and machine learning environment as it created red flags in their application development processes. The existing solution worked in a test environment, but failed in a machine learning and AI-driven analysis environment as it would have exposed live data and created a breach risk from either accidental exposure, insider threats, or external attacks.
Sensitive data would have to be protected yet kept in a format that would allow it to be processed by machine learning and AI based applications.
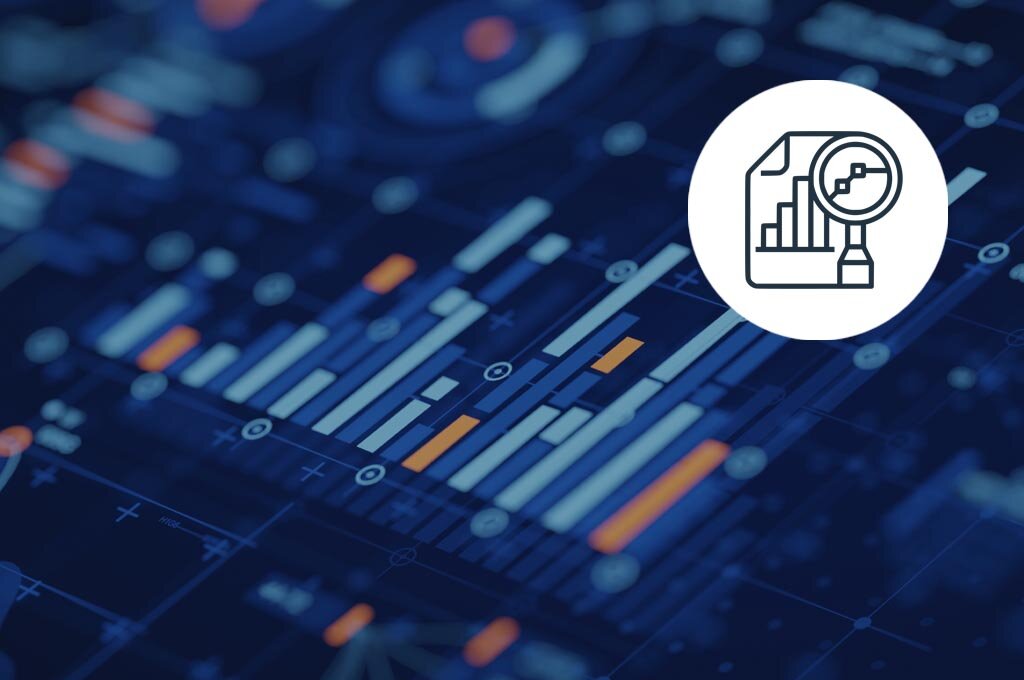
Zero Trust Data Transfer and Move to SaaS Applications
Their customer data hub contained a mix of non-sensitive data and PII which was managed by offshore teams. In keeping with a zero trust methodology, they wanted to protect all sensitive data whenever possible in order to reduce the risk of both internal and external data leaks.
In addition, they were onboarding SaaS applications in the near future, which also required a solution that would prevent PII from unnecessarily being left exposed in or while being transferred to the cloud. Unfortunately, traditional controls that come with modern cloud platforms tend to be from a prior generation of data-atrest and data-in-motion access controls and perimeter-based controls. In many cases, these controls only protect the data after it has already entered the cloud, which presents a major security gap.
Further complicating the matter, they wanted to move a merged IT infrastructure (from 13 companies) from a data-center-centric model to a cloud-distributed model, which required a much more powerful data discovery mechanism than what they had been running.
Continuous & Agentless Data Discovery
The first step in plugging data security gaps and eliminating risk is knowing where all sensitive data is stored. We replaced the existing discovery technologies they had with an automated continuous solution that is able to discovery unknown repositories and enables a far more efficient and effective data discovery process. This means not just being able to locate sensitive data, but also knowing what the data is used for, where it’s used, and which applications are processing it. This can all now be done on an automated basis across the enterprise and up into the cloud ecosystems. This gave them a very clear picture of where risks are and where additional controls were needed in order to meet new compliance and risk reduction mandates.
Additionally, the data discovery solution is agentless, meaning it puts very little burden on servers so high volumes of data can be scanned in a relatively short amount of time.
Scalable, end-to-end Data Protection
The issue of sensitive data mixed in with non-sensitive data in free form text fields was a serious challenge for the company. Our solution met that challenge by automatically locating sensitive data elements within the freeform text field, and applying the appropriate form of protection based on their policies. If for example, the last four digits of SSNs or a bank account number don’t need to be protected, they can be left in clear text, while the rest is pseudonymized or masked. Even where protection has been applied, the data can be kept in a recognizable format to enable machine-learning and sentiment analysis.
Next, we removed siloed data protection solutions to create a singular, continuous, and iterative workflow within the organization. We instrumented data security as a service into their DevOps program and enabled the business to consume protected production data with data-centric security applied in these live environments. We protect data endto-end from acquisition to operations to data science platforms in any cloud. Our cloudnative, DevOps friendly approach where infrastructure could be run on Kubernetes solved this issue, which we demonstrated in the POC.
This holistic approach reduces the exposure of personal data, allows offshore data management without exposing it, and yet still creates the ability to handle and process all this data, enabling very interesting analytics and insights for all sorts of predictive analytics and customer sentiment.
Transparent integration
One of the key applications in scope was Informatica MDM/360 which has no APIs to integrate third party encryption. In contrast to the API approach of their existing solution, our solution could be implemented in a faction of time with a fraction of the effort.
Our data security platform allows “snap-in” integration to processes identified as high risk during data discovery. In many cases, data protection can be achieved without having to change the respective application. Transparent integration is also available for files, streams, databases and pipes ranging from JDBC intercepts to native integration options (i.e., Apache Kafka). This allows sensitive data to be effectively secured on the fly at capture and therefore over its entire lifecycle.
Like many firms on a transformation journey to the cloud, DevOps, machine intelligence, and automation, the backdrop of privacy regulations and breach risk can be a huge roadblock – we are enabling this insurer to leap forward and continue on their growth journey to the top of the insurance rankings and move up the Fortune 250 leaderboard.
Agile Protection for Data Privacy Regulations
Data is protected end to end in accordance with NACHA as well as many other data privacy regulations as strong data protection is a common requirement. To ensure sustainability, our scalable solution can easily be configured to include additional data elements that may come under the scope of future regulations.
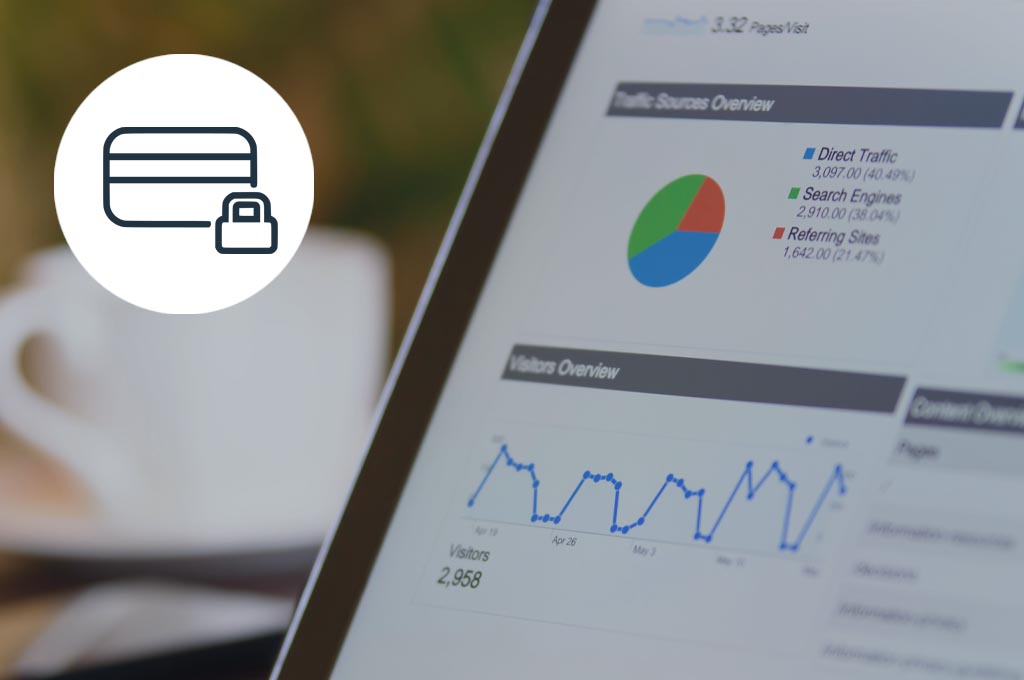
More Effective Fraud Detection and Business Insights
One of the greatest benefits to format-preserving data protection is that data science teams can make use of much larger data sets without exposing sensitive data. This means they can more effectively gain valuable insights, such as detecting fraud.
Efficient Data Governance and Risk Reduction
One of the major challenges for data governance is understanding the data landscape and to determining whether discovered data should be classified as sensitive and valuable, and therefore requiring risk mitigation. This process is now entirely automated which saves a great deal of time and resources and reduces risk.
They now have a clear picture of how their data is being stored, processed, and shared in near real-time. They can automatically discover and analyze all usage of data and its lineage without having to rely upon pre-existing knowledge of the presence or location of data.
With this discovery knowledge, they can create effective protection policies and implement appropriate security controls. They can identify sensitive data, protect it properly, then monitor ongoing changes in the data ecosystem.
Our Discovery and Classification solution enforces better privacy, security, and governance measures by creating a Master Data Catalog inventory. Linking all the pieces into a comprehensive informational picture makes it easier to identify compliance risk and manage data subject access requests—including the right to erasure, update, or share data changes.
"Comforte offers impeccable support which has helped make the implementation process go very smoothly. Whenever we reach out to them we get a prompt response from experienced engineers who can quickly diagnose and solve any issues so things can keep moving forward."
– Data Security Engineer at Major US Insurance Provider